Sourced by Dr.Vanessa Panettieri, Editor (Educational), AFOMP Pulse
In the past six months the AFOMP journals have published a collection of very exciting papers in Medical Physics ranging from tumor definition to quality assurance. For this edition of PULSE we have selected a collection that focuses on three current topics.
The first section will look at the use of Artificial Intelligence(AI) applications ranging from tumor classification, segmentation and quality assurance. As we can see from the increasing number of publications in all the main scientific journals and presentations at conferences AI is becoming one of the most transformative technologies in our field, rapidly changing the way diagnostic imaging and radiotherapy tasks, traditionally relying on human skills, are now approached. AI tasks (often applied using machine learning (ML) or deep learning (DL) methodologies) have the increasing potential to automate laborious tasks, improve workflow, and enhance efficiency in Medical Physics and other processes in the overall patient treatment chain.
In the second part we will explore work looking at differences in skin dose when techniques such as Intensity Modulated Radiation Therapy (IMRT) and Volumetric Modulated Arc Therapy are used on two different linear accelerator technologies. Finally for our newly created “How to?” section we will take you through a practical approach to assess the focal spot alignment in clinical linear accelerators.
Happy reading!
All papers contributions are kindly provided by Dr Taylah Brennen and Sadia Aftab, Medical Physicists, Peter MacCallum Cancer Centre
1) Focus on: Artificial Intelligence
This paper presented by Pal et al on the Journal of Medical Physics explores the use of radiomic feature detection in MRI brain image datasets to enable machine learning based tumor classification. The authors have shown how their unique hybrid technique can outperform other currently published methods of tumor classification in metrics such as accuracy, sensitivity, specificity and F1 score. This work shows promise for the integration of machine learning in the space of medical imaging, and it identifies that the image preprocessing step prior to model development can have a large impact on the accuracy and reliability of feature identification. It highlights how the use of freely available software can be harnessed to create models to predict tumor classifications, resulting in an automated way to identify key characteristics of a tumor that may be indicative of a poor prognosis. The ultimate limitation to this introduction of AI and machine learning models is access to high quality data. It is becoming clear that to create accurate and robust models it is essential for medical physicists around the world to collaborate, create and share data in easy and ethical ways.
Moving on to the second publication as AI tools have proven to be at the forefront of new features being explored, it is only fitting that in the world of Medical Physics we also want to explore the capabilities of AI for quality assurance (QA). This paper presented by Salahuddin et al provides us all with a glimpse into the possibilities of integrating AI into our routine QA for linear accelerators. The paper moves towards addressing the question “what can we do to prevent failures in QA before they arise?”. The answer or part thereof, could indeed be the integration of AI into our routine clinical QA tasks such as the Winston-Lutz test. With departments growing busier and with more complex treatments such as stereotactic ablative body irradiation (SABR) becoming more standardly used there is a need to ensure higher levels of machines precision, while minimising their down time. This paper has highlighted the promising capabilities of being able to utilise a range of machine learning models to accurately identify and help predict the failure modes for isocentre stability. Ultimately this methodology could allow for better trend analysis and provide the means for early error prediction, ensuring complex and high dose treatments such as SABR can be delivered with confidence and minimal treatment time interruptions.
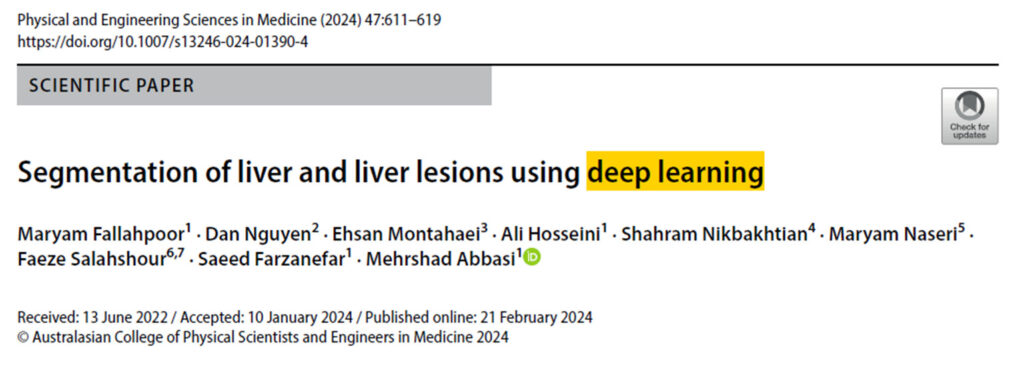
The final contribution explores the application of AI in auto-contouring a tool now starting to be widely used in clinics around the world. In particular, this manuscript recently published in the journal of Physical and Engineering Sciences in Medicine analysed the application of AI and deep learning (DL) techniques for the segmentation of liver and liver lesions using magnetic resonance imaging (MRI) scans. The authors discuss the advantages of using AI in organ segmentation highlighting the potential applications of this automated approach in nuclear medicine, image interpretation, and mass image processing. Overall, the study demonstrates the potential of DL techniques for accurate liver and liver lesion segmentation in MRI scans, which can have various clinical and research applications. A U-Net architecture-based DL model called Isensee 2017 was used to perform the segmentation. The results showed that the DL model was able to effectively segment the liver and liver lesions in both T1-weighted (T1w) and T2-weighted (T2w) MRI images, and that the performance of the DL model was superior to previous studies that used ML approaches. The authors also highlighted the challenges and limitations of preparing MRI data for DL training. Furthermore, the segmentation results can also be used for attenuation correction in PET/MRI scanners. Attenuation correction is an important step in PET imaging, and accurate segmentation of the liver that can help improve the accuracy of this correction process. In my view, this work brings potential applications in radiotherapy medical physics. Accurate segmentation of the liver and liver lesions is crucial for dosimetry in radiation therapy, as it allows precise targeting of the tumor and sparing of healthy tissues, as it can be challenging to isolate lesions from normal liver.
2) Focus on : Treatment planning studies
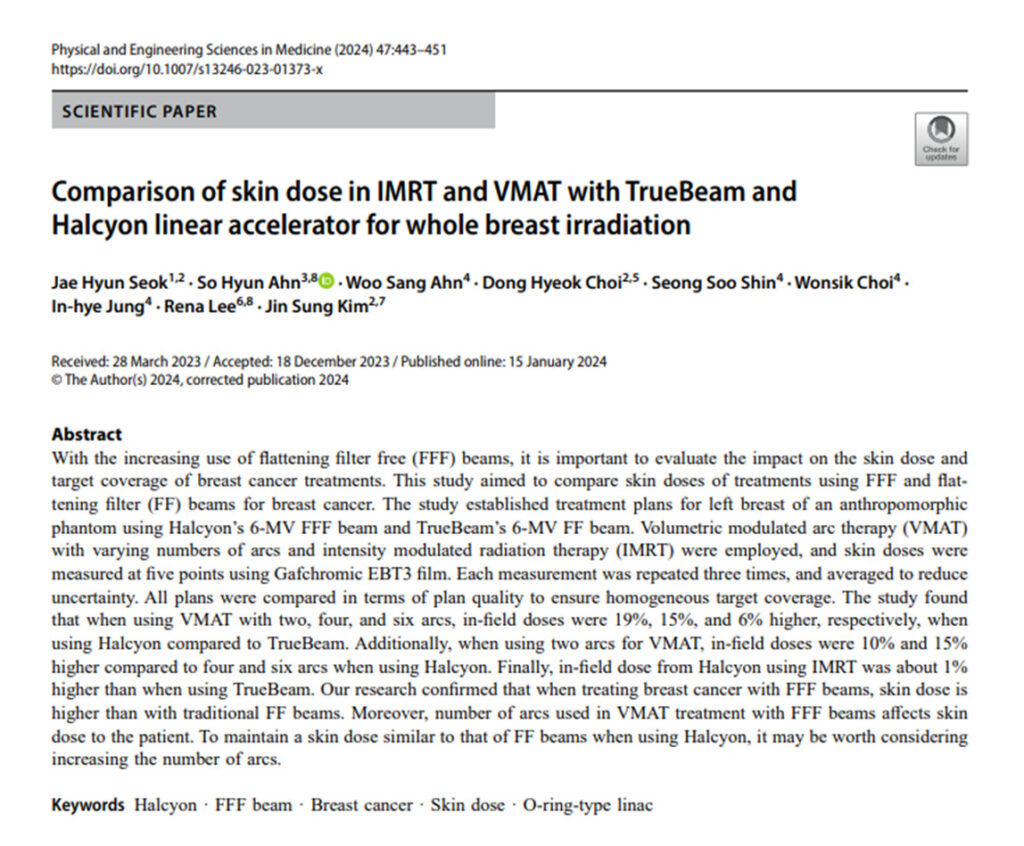
With the number of cancer patients requiring treatment ever increasing, technology and treatment techniques have had to adapt to enable fast treatment times while still upholding a high standard of care. The paper presented by Seoket al has shown some of the considerations that need to be made when employing flattening filter free (FFF) beams for treatment. Though they provide a faster delivery due to a higher dose rate being employed the implications of using FFF beams, such as removal of beam hardening, must be considered in the context of all treatment sites. For a site such as the breast it is imperative to ensure appropriate skin doses are being adhered to in order to minimise the likelihood of skin reactions and poor cosmetic outcomes for patients. It was found in this study that, as expected, due to the lower energy FFF beam, that skin doses increased quite considerably compared to flattened beams. With this knowledge the group compared doses for a range of techniques and concluded that skin dose was dependent on the number of VMAT arcs employed. With inverse planned techniques becoming more popular in the breast radiation therapy space it seems feasible for centres to employ VMAT techniques using FFF beams in such a way that the skin dose can be comparable to that of a flattened beam. Though we all must remember – increasing the arc number comes at a cost by increasing treatment – so it is important to ask ourselves: is the time gained from using a FFF beam for treatment greater than that added by using multiple VMAT arcs to ensure adequate skin doses?
3) Focus on : How to?
This section provides practical information on tests and procedures that can be used in the clinic to ensure equipment accuracy and precision.
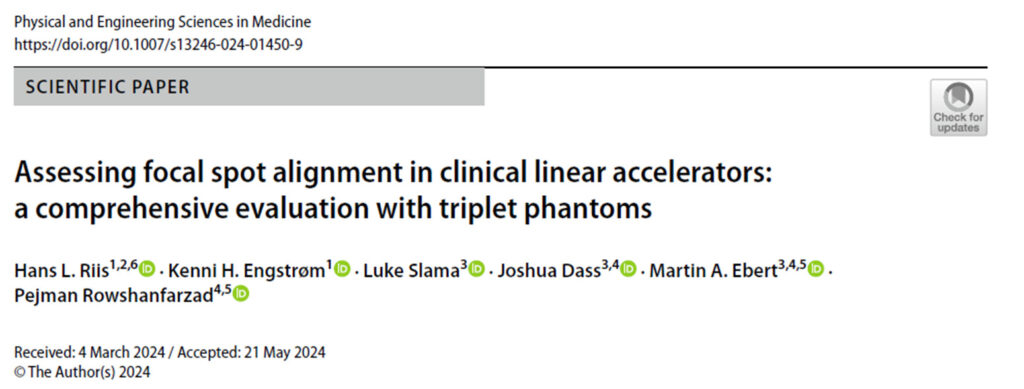
This interesting paper recently published in Physical and Engineering Sciences in Medicine evaluated the focal spot position (FSP) in clinical linear accelerators (linacs) and analysed the ability of different phantoms to detect effects on patient plan delivery related to FSP. The focal spot position is an important parameter to evaluate the beam delivery precision and stability of a linac in terms of beam steering.
In the study the authors conducted comprehensive FSP measurements using a rigid phantom holding two ball-bearing at two different distances, by acquiring images on the EPID panel. They also analysed the acquired data and evaluated the performance of alternative phantoms, including the PTW STARCHECK phantom and the SNC ArcCHECK phantom, in detecting FSP effects on patient plan delivery. This paper also discusses the limitations and challenges in measuring FSP, capability of any phantom to detect a subsequent impact on the accuracy of patient plan delivery and provides insights into the factors that can affect FSP, such as beam energy, dose rate, monitor units, gantry angle and sag. Overall, this paper contributes to the understanding of FSP and provides insights into its clinical implications. FSP appears to be less relevant than central axis position (CAP) at the isocentre. While the regular QA program focuses on beam symmetry, flatness, field size and radiation isocentre location, it is recommended by this study to establish the tolerance levels for CAP measured with gantry rotation because CAP contains the FSP and sag effects. Any deviation of CAP from tolerance levels, potential issues related to beam steering (FSP) and stability can be identified and addressed, leading to improved treatment outcomes for patients.