Go to AFOMP Pulse Contents/Index Page
From Pixels to Patients: How developments in the gaming and smartphone industry are shaping Radiation Oncology
(Dr. Michael Douglass, Principal Medical Physicist, Royal Adelaide Hospital, Australia)
As a researcher in the field of medical physics, I have always been fascinated by the intersection of consumer technologies and radiation oncology. In a recent presentation to the International Organisation of Medical Physics (IOMP), I discussed the influence of developments in the gaming, movie, and smartphone industries on radiation oncology. These industries have pushed the development of GPUs and other specialised technologies, leading to various advancements that are now improving radiation oncology.
The history of video games is a fascinating one. Two iconic titles from the early era of arcade games were “Pong” and “Space Invaders”. Then home video game consoles, such as the Nintendo entertainment system and Sega Genesis started to appear in the 1980s. In the 1990s, 3D games consoles like the Nintendo® 64 and PlayStation® required more powerful graphics processing. By the 2000s, high-quality games demanded the introduction of dedicated graphics processing units, or GPUs, by manufacturers like Nvidia® and AMD®. By the 2010s, GPUs had become general-purpose high-performance processing tools for accelerating code performance, including scientific applications. GPU manufacturers then started optimising GPU cards specifically for machine learning applications, which enabled the rapid advancement of this field. The demand for high-quality graphics in games has driven the development of powerful and general-purpose GPUs. These GPUs have been optimised for AI and deep learning applications, leading to significant advances in these fields. By the 2020s, the GPU had become the heart of AI and machine learning, with specialised GPU architectures being developed for machine learning, such as the Nvidia® tensor core and Google® tensor Processing Unit.
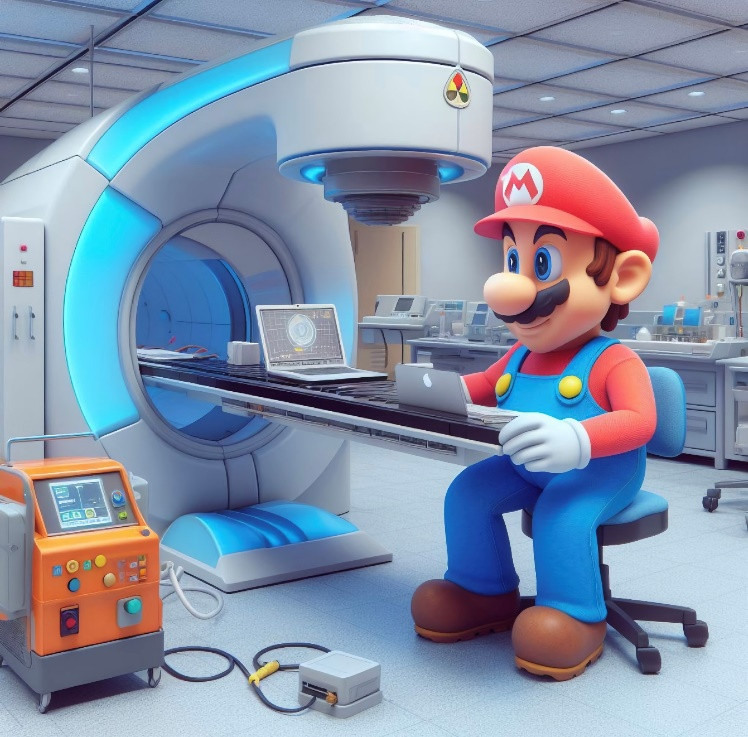
One example of how these advancements in GPU technology have improved radiation oncology is deep learning segmentation. This uses convolutional neural networks to automatically segment organs at risk and targets in treatment planning systems. This has led to more consistent and accurate contours for patient plans, saving radiation oncologists a significant amount of time. This type of application is likely to expand into more general-purpose segmentation models. The Meta® segment anything model (SAM) is an example of a general purpose, open-source machine learning model that can segment almost any image data, including medical data. This model has the potential to be a direction that radiation oncology heads in, where one general-purpose model can be used to segment various regions of the body, rather than having to train machine learning models specifically for cranial sites or abdominal sites, for example.
Another area where GPU technology has been beneficial is in dose calculations in treatment planning systems. Major vendors such as Electa®, Varian®, and Raysearch Laboratories® are now using GPU acceleration in their dose calculation engines, allowing for highly optimised treatment plans and accurate Monte Carlo dose calculations in seconds to minutes. This has led to rapid optimisation of treatment plans, meaning that more iterations or optimisation steps can be made in the same amount of time, leading to the ability to achieve a more optimal treatment plan in a shorter amount of time. In addition, being able to calculate predicted radiation dose distribution using Monte Carlo in a clinically acceptable time means there are fewer uncertainties in the delivered dose due to inhomogeneities. This is because Monte Carlo dose calculations are considered the gold standard for dose calculations, as they more accurately model the transport of radiation through matter.
Clinical workflows can benefit from the assistance of large language models, which have become more prevalent in our everyday lives. For example, GitHub co-pilot, a variation of GPT trained on programming and scripting data, can aid in writing scripts for treatment planning systems resulting in more efficient planning workflows. Large language models may also help summarise vast amounts of patient medical data in various forms which may assist doctors make more informed clinical decisions.
In addition to GPU technology, the video game industry has also given us virtual reality technologies. These advances will lead to more intuitive methods of visualising medical data and be important for various clinical techniques, such as deep inspiration breath-hold and guiding the patient to reach the optimal inhalation limit. Virtual reality could also be important for education, both for staff and patients.
The movie industry has led to advancements in visual effects. Data synthesis using techniques adopted from the film and movie industry can be used to generate enormous amounts of training data automatically and rapidly. This allows machine learning models to be trained on large amounts of synthetic training data and then fine-tuned on real data to improve accuracy and robustness of predictions. This can be particularly useful in radiation oncology, where access to real clinical data may be limited or require ethics applications. Some examples of papers where synthetic training data have been used include for automotive applications (Alhaija et. al., 2017), synthetic glioblastomas generated in patient CT data (Birgit et. al., 2018), and automatically segmenting nanoparticle microscopy images (Mill et. al., 2020).
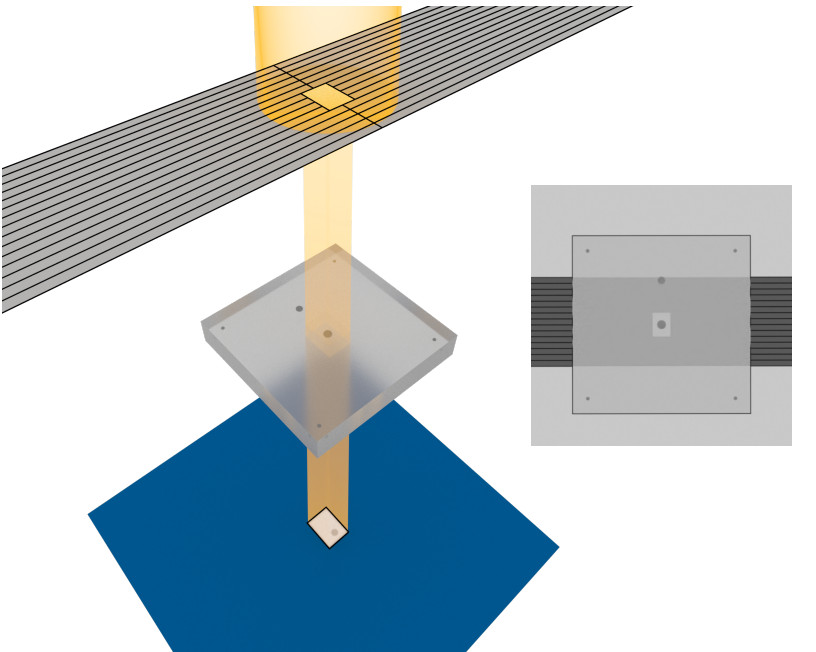
Smartphones, with their advanced sensors and 3D scanning technologies, have led to faster, high-quality, and more intuitive methods of collecting and visualising medical data. This could lead to new possibilities for 3D printing medical devices and boluses for radiation oncology. Smartphones have led to significant advancements in the field of 3D scanning, making it more cost-effective, radiation-free, and faster to reconstruct. These technologies can benefit patients through 3D printing of customised medical devices and bolus, radiation-free simulations for specialised treatments such as total body irradiation and total skin electron therapy without the need for a CT scan. This has the potential to improve patient care and make treatments more readily available.
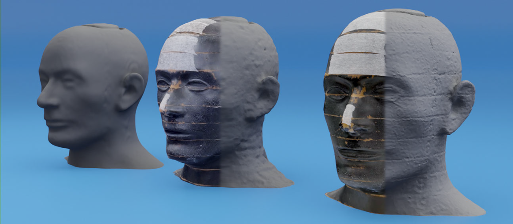
Source: M. Douglass, J Med Radiat Sci. 69 (2022) 139–142
Developments in GPU, 3D modelling software, and scanning hardware, driven by the movie, smartphone, and gaming industries, have reduced costs and enabled access to various research and clinical tools for radiation oncology. These advancements are leading to rapid development and providing many opportunities to explore novel technologies as medical physicists and clinicians. The future is likely to see even faster and more powerful GPUs, real-time visualisation of patient treatments, and the incorporation of large language models into clinical workflows.